Table of Contents
KanBo – The Pharma-Focused Work Coordination Maestro
Experience ultimate task alignment, communication and collaboration
Trusted globally, KanBo, bridges the gap between management and engineering in complex pharmaceutical organizations. Seamless coordination, advanced project planning, and outstanding leadership are made possible through our versatile software. Stride toward your mission-critical goals with superior collaboration and communication.
KanBo Data Science Industrialization, Machine Learning Engineering Manager Challenge: Streamlining Collaborative Data Science Pipelines
What do you need to know about this challenge?
In the realm of data science industrialization, the Data Science Industrialization, Machine Learning Engineering Manager faces the complex task of bringing together diverse data sets and analytical models across various departments. This challenge requires managing the integration of machine learning workflows into business infrastructure, ensuring scalable and automated processes that are robust and effective.
What can you do with KanBo to solve this challenge?
KanBo features several functionalities that can address the hurdles faced by managers in creating collaborative data science pipelines:
- Pipeline Structuring: Use KanBo card to represent each step of the data science pipeline, encompassing tasks such as data preprocessing, modeling, evaluation, and deployment.

- Workflow Automation: Take advantage of KanBo Gantt Chart view to plan and visualize the stages of pipeline development and track the progress of each card against a timeline.
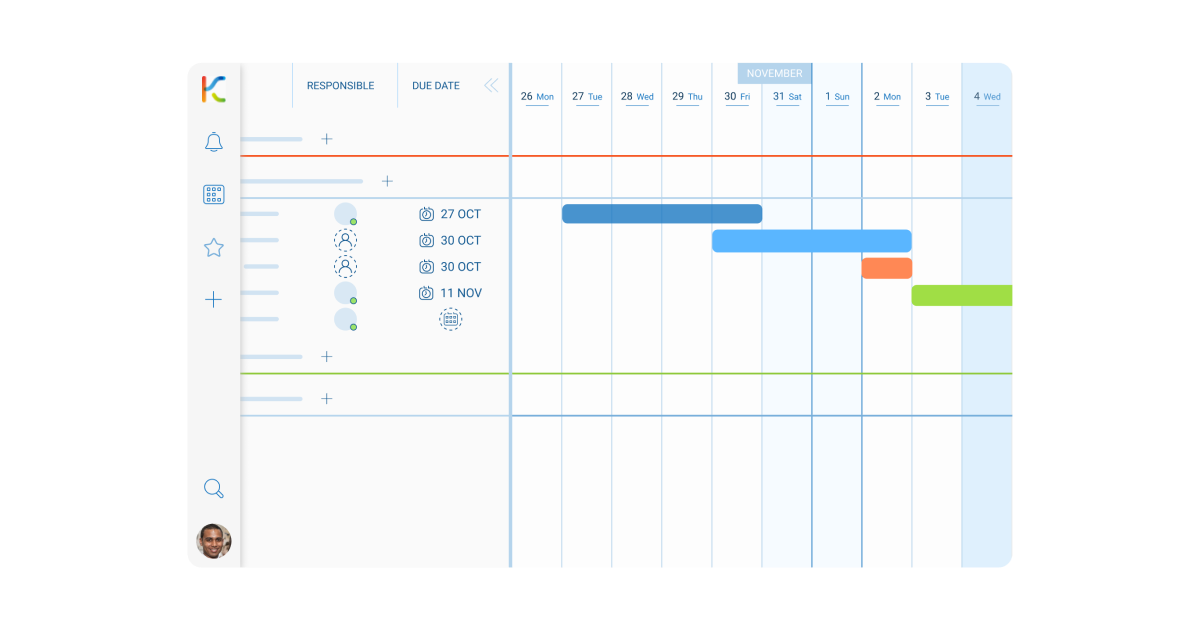
- Real-time Collaboration: Utilize the KanBo activity stream to keep everyone informed and engaged with each update on a machine learning workflow, providing transparency across the project lifecycle.
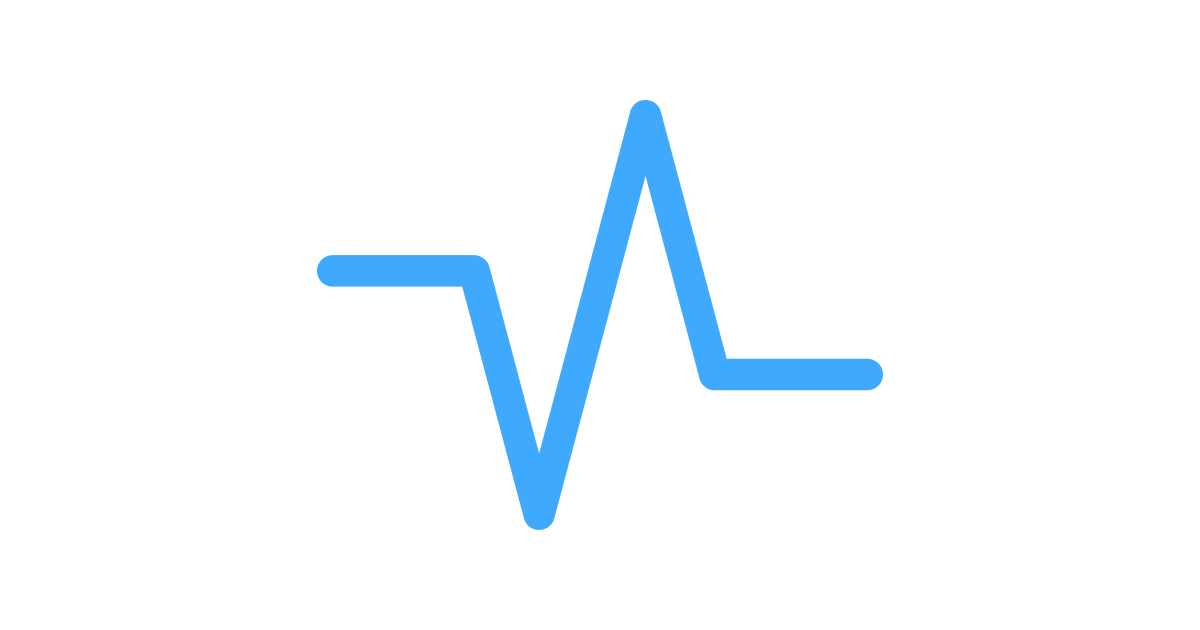
- Code and Model Repository Integration: Incorporate document sources into your cards to organize and reference scripts, Jupyter notebooks, as well as trained models for easy access within your data science team.

- Continuous Monitoring: Apply card activity stream to each machine learning card for detailed tracking of modifications and contributions, creating a log for auditing and review purposes.
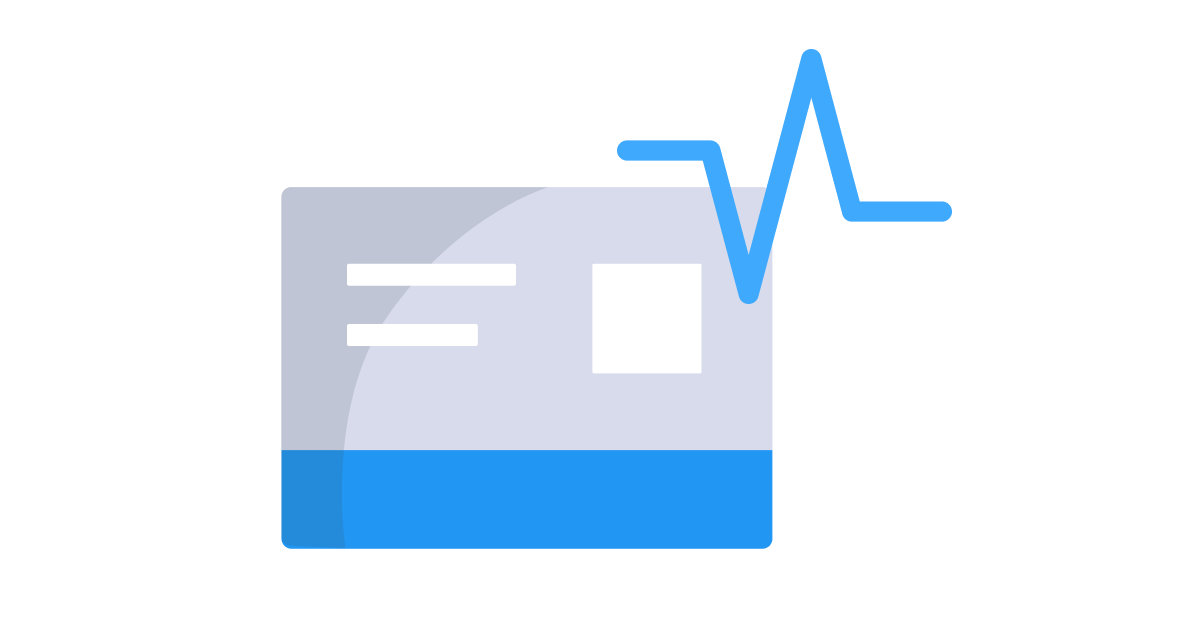
- Scalable Execution Tracking: Use the KanBo filter cards feature to sift through pipeline components based on various criteria such as model performance metrics or data quality indicators.
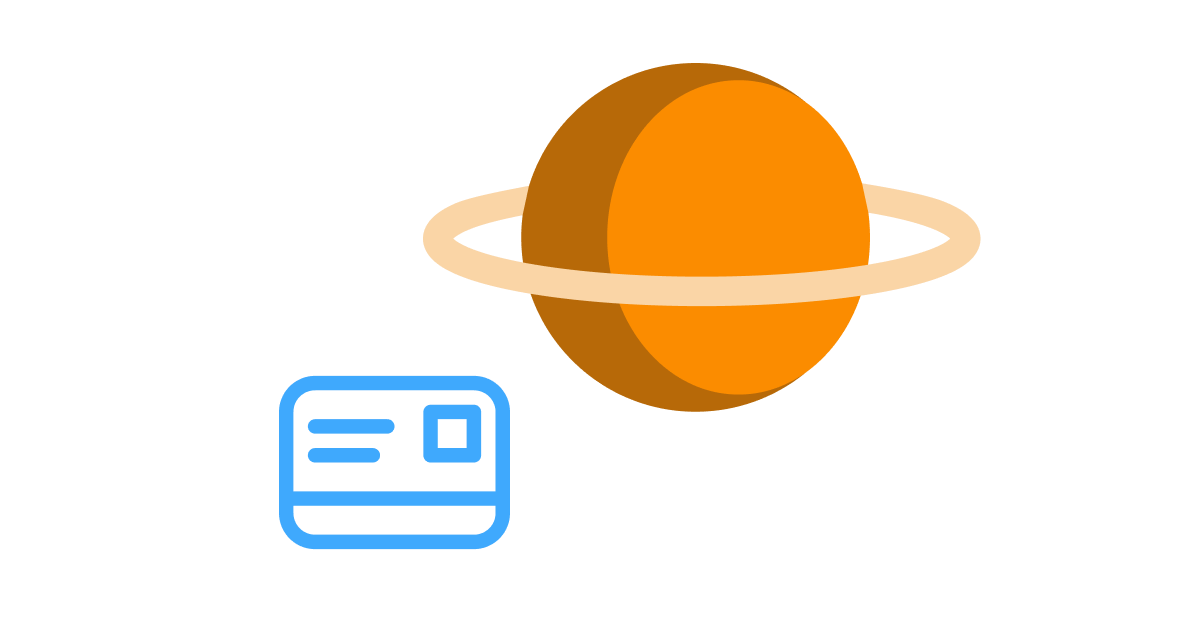
- Efficient Resource Allocation: Organize your team's capabilities and pipeline components using KanBo grouping, enabling the clear assignment and management of responsibilities.
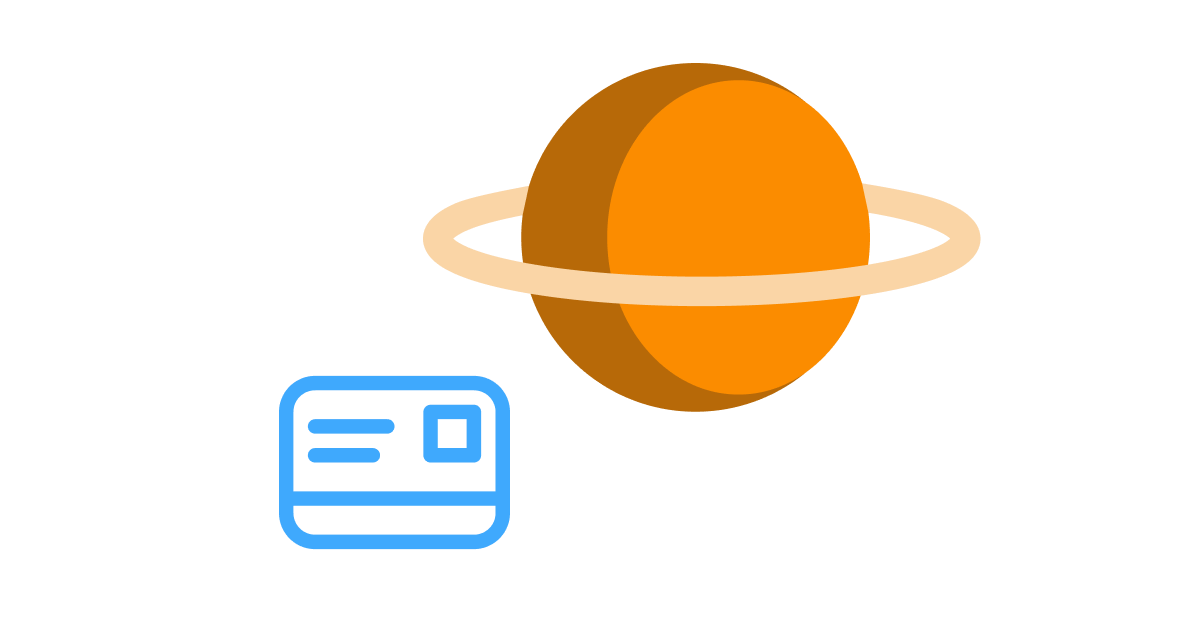
What can you expect after solving this challenge?
By effectively leveraging KanBo features to tackle the collaboration challenge within the industrialization of data science, you will achieve an organized and transparent workflow conducive to innovation and efficiency. Expect improved coordination among team members, leading to a proactive response to data pipeline issues and needs. Enhanced traceability in terms of contributions and pipeline evolution will result in higher code quality and reproducibility. Over time, leveraging these solutions will establish a solid foundation for deploying robust machine learning models faster and more reliably, ensuring these solutions remain scalable and flexible to adapt to future demands and technological shifts in data science industrialization.
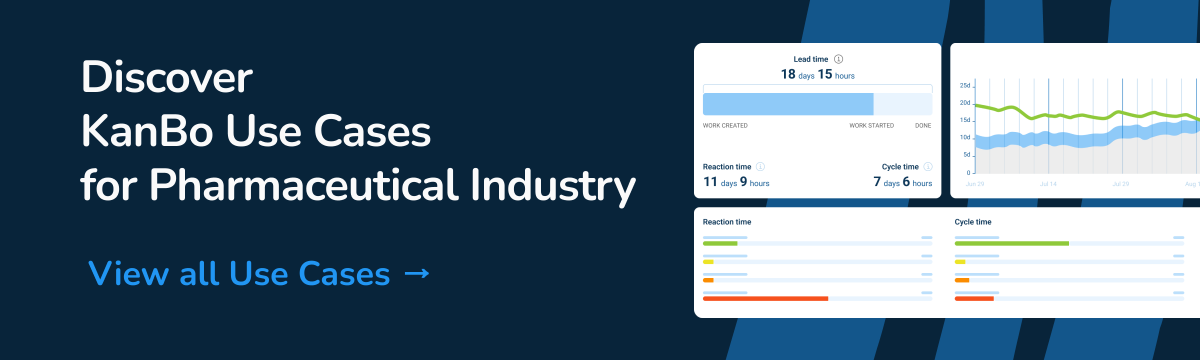
Table of Contents
KanBo – The Pharma-Focused Work Coordination Maestro
Experience ultimate task alignment, communication and collaboration
Trusted globally, KanBo, bridges the gap between management and engineering in complex pharmaceutical organizations. Seamless coordination, advanced project planning, and outstanding leadership are made possible through our versatile software. Stride toward your mission-critical goals with superior collaboration and communication.
KanBo Data Science Industrialization, Machine Learning Engineering Manager Challenge: Streamlining Collaborative Data Science Pipelines
What do you need to know about this challenge?
In the realm of data science industrialization, the Data Science Industrialization, Machine Learning Engineering Manager faces the complex task of bringing together diverse data sets and analytical models across various departments. This challenge requires managing the integration of machine learning workflows into business infrastructure, ensuring scalable and automated processes that are robust and effective.
What can you do with KanBo to solve this challenge?
KanBo features several functionalities that can address the hurdles faced by managers in creating collaborative data science pipelines:
- Pipeline Structuring: Use KanBo card to represent each step of the data science pipeline, encompassing tasks such as data preprocessing, modeling, evaluation, and deployment.

- Workflow Automation: Take advantage of KanBo Gantt Chart view to plan and visualize the stages of pipeline development and track the progress of each card against a timeline.
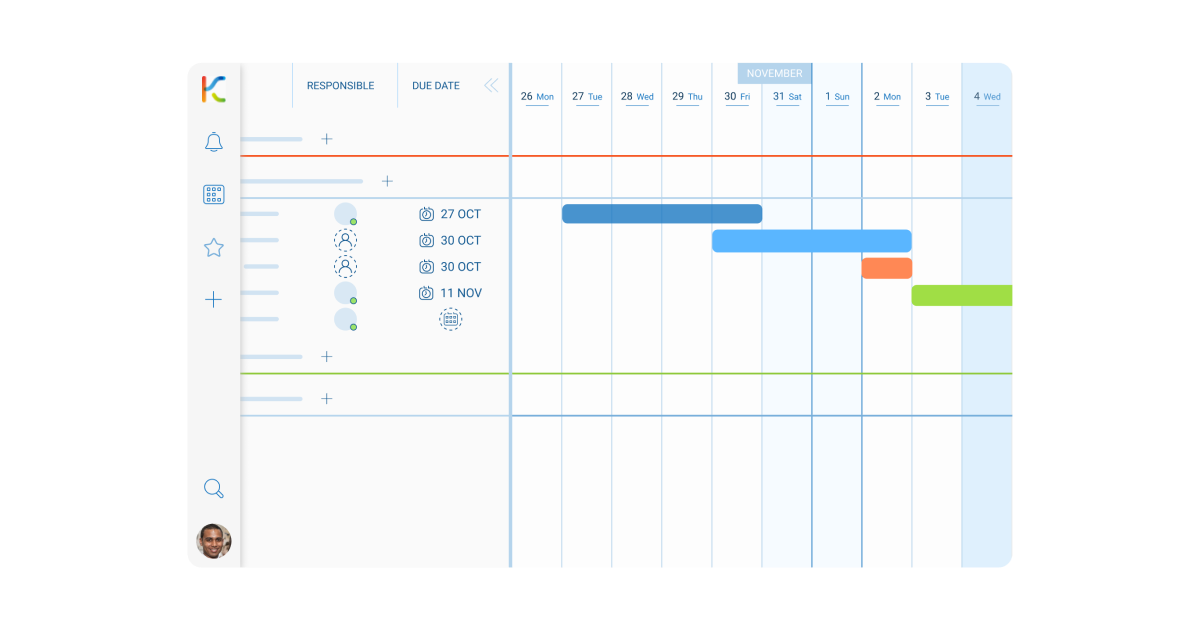
- Real-time Collaboration: Utilize the KanBo activity stream to keep everyone informed and engaged with each update on a machine learning workflow, providing transparency across the project lifecycle.
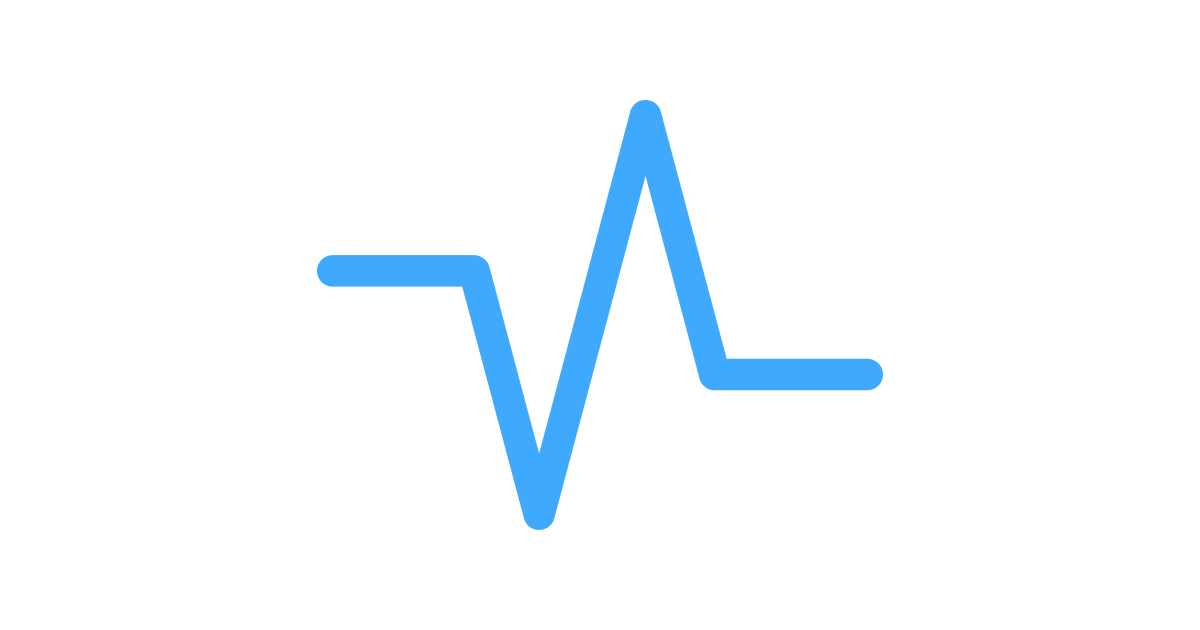
- Code and Model Repository Integration: Incorporate document sources into your cards to organize and reference scripts, Jupyter notebooks, as well as trained models for easy access within your data science team.

- Continuous Monitoring: Apply card activity stream to each machine learning card for detailed tracking of modifications and contributions, creating a log for auditing and review purposes.
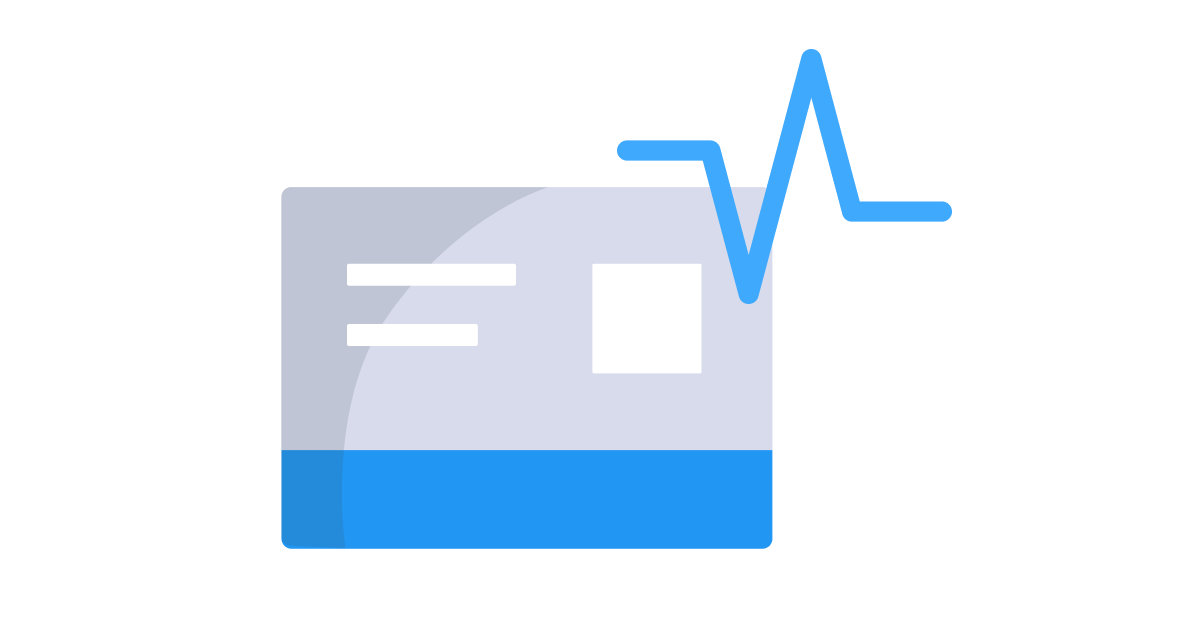
- Scalable Execution Tracking: Use the KanBo filter cards feature to sift through pipeline components based on various criteria such as model performance metrics or data quality indicators.
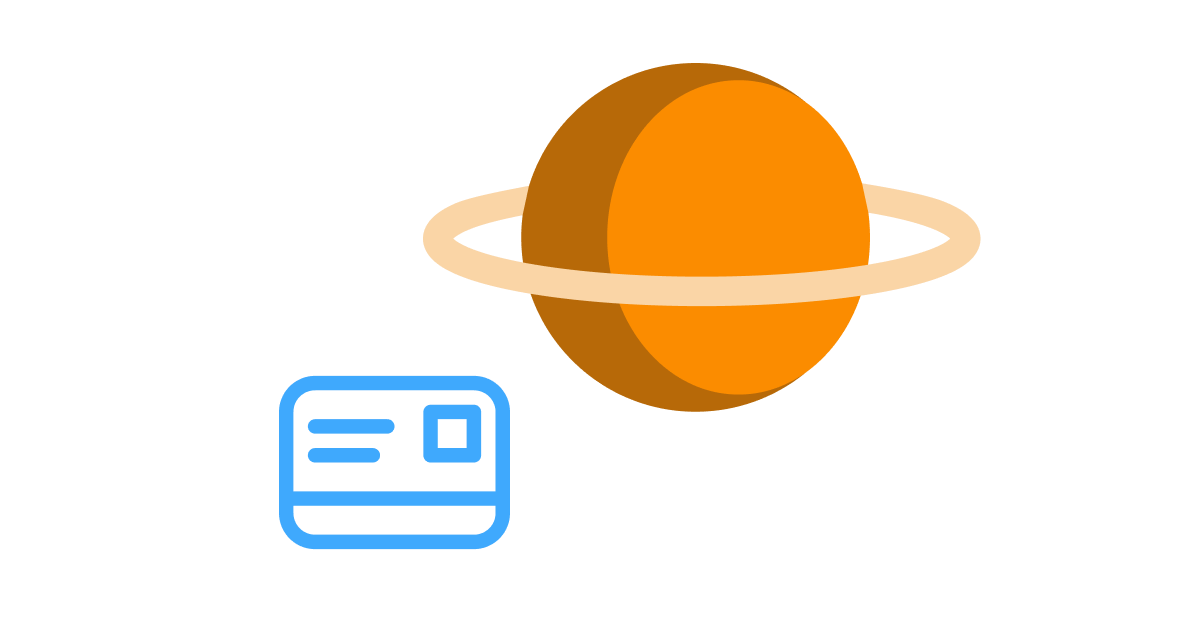
- Efficient Resource Allocation: Organize your team's capabilities and pipeline components using KanBo grouping, enabling the clear assignment and management of responsibilities.
What can you expect after solving this challenge?
By effectively leveraging KanBo features to tackle the collaboration challenge within the industrialization of data science, you will achieve an organized and transparent workflow conducive to innovation and efficiency. Expect improved coordination among team members, leading to a proactive response to data pipeline issues and needs. Enhanced traceability in terms of contributions and pipeline evolution will result in higher code quality and reproducibility. Over time, leveraging these solutions will establish a solid foundation for deploying robust machine learning models faster and more reliably, ensuring these solutions remain scalable and flexible to adapt to future demands and technological shifts in data science industrialization.
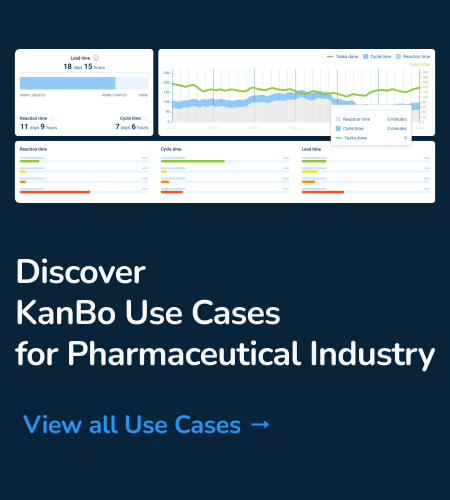